Non-IID data and Continual Learning processes in Federated Learning: A long road ahead
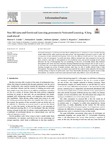
Use this link to cite
http://hdl.handle.net/2183/31818Collections
- GI-GAC - Artigos [181]
Metadata
Show full item recordTitle
Non-IID data and Continual Learning processes in Federated Learning: A long road aheadAuthor(s)
Date
2022Citation
M. F. Criado, F. E. Casado, R. Iglesias, C. V. Regueiro, y S. Barro, «Non-IID data and Continual Learning processes in Federated Learning: A long road ahead», Information Fusion, vol. 88, pp. 263-280, dic. 2022, doi: 10.1016/j.inffus.2022.07.024.
Abstract
[Abstract] Federated Learning is a novel framework that allows multiple devices or institutions to train a machine learning model collaboratively while preserving their data private. This decentralized approach is prone to suffer the consequences of data statistical heterogeneity, both across the different entities and over time, which may lead to a lack of convergence. To avoid such issues, different methods have been proposed in the past few years. However, data may be heterogeneous in lots of different ways, and current proposals do not always determine the kind of heterogeneity they are considering. In this work, we formally classify data statistical heterogeneity and review the most remarkable learning Federated Learning strategies that are able to face it. At the same time, we introduce approaches from other machine learning frameworks. In particular, Continual Learning strategies are worthy of special attention, since they are able to handle habitual kinds of data heterogeneity. Throughout this paper, we present many methods that could be easily adapted to the Federated Learning settings to improve its performance. Apart from theoretically discussing the negative impact of data heterogeneity, we examine it and show some empirical results using different types of non-IID data.
Keywords
Federated learning
Data heterogeneity
Non-IID data
Concept drift
Distributed learning
Continual learning
Data heterogeneity
Non-IID data
Concept drift
Distributed learning
Continual learning
Editor version
Rights
Atribución 3.0 España
ISSN
1566-2535
Related items
Showing items related by title, author, creator and subject.
-
Una integración a sistemas de gestión de aprendizaje en estándares de un sistema barra-bola
Montoro, Alicia; Ruano Ruano, Ildefonso; Estévez, Elisabet; Gómez Ortega, Juan; Gámez García, Javier (Universidade da Coruña, Servizo de Publicacións, 2021)[Resumen] Los laboratorios de tipo online tienen cada vez más aceptación dentro de la educación universitaria relacionada con las ciencias, tecnologías, ingenierías y matemáticas (CTIM o STEM en inglés), donde el trabajo ... -
Análisis de un robot abierto de bajo coste para docencia de aprendizaje automático
Bes Carreras, Jorge; García-Barcos, Javier; Martínez-Cantín, Rubén (Universidade da Coruña. Servizo de Publicacións, 2023)[Resumen] En este trabajo estudiamos el comportamiento, ventajas e inconvenientes del robot Trifinger para la docencia de estudiantes de ingeniería y automática. El robot Trifinger es un robot de diseño abierto a nivel ... -
Lifelong Learning and Personal Learning Environments: a Productive Symbiosis in Higher Education
García-Martínez, José Antonio; González-Sanmamed, Mercedes; Muñoz-Carril, Pablo-César (Universidad Complutense de Madrid, 2023-01-09)[Abstract] The aim of this study was to analyze lifelong learning (LLL) and its relationship with the educational approach of the personal learning environment (PLE). In the last year students do various degrees at a ...