FacialSCDnet: A deep learning approach for the estimation of subject-to-camera distance in facial photographs
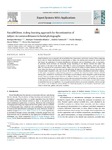
Use this link to cite
http://hdl.handle.net/2183/31816
Except where otherwise noted, this item's license is described as Atribución-NoComercial-SinDerivadas 3.0 España
Collections
- Investigación (FIC) [1685]
Metadata
Show full item recordTitle
FacialSCDnet: A deep learning approach for the estimation of subject-to-camera distance in facial photographsAuthor(s)
Date
2022Citation
E. Bermejo, E. Fernandez-Blanco, A. Valsecchi, P. Mesejo, O. Ibáñez, y K. Imaizumi, «FacialSCDnet: A deep learning approach for the estimation of subject-to-camera distance in facial photographs», Expert Systems with Applications, vol. 210, 30 dic. 2022, doi: 10.1016/j.eswa.2022.118457.
Abstract
[Abstract]: Facial biometrics play an essential role in the fields of law enforcement and forensic sciences. When comparing facial traits for human identification in photographs or videos, the analysis must account for several factors that impair the application of common identification techniques, such as illumination, pose, or expression. In particular, facial attributes can drastically change depending on the distance between the subject and the camera at the time of the picture. This effect is known as perspective distortion, which can severely affect the outcome of the comparative analysis. Hence, knowing the subject-to-camera distance of the original scene where the photograph was taken can help determine the degree of distortion, improve the accuracy of computer-aided recognition tools, and increase the reliability of human identification and further analyses. In this paper, we propose a deep learning approach to estimate the subject-to-camera distance of facial photographs: FacialSCDnet. Furthermore, we introduce a novel evaluation metric designed to guide the learning process, based on changes in facial distortion at different distances. To validate our proposal, we collected a novel dataset of facial photographs taken at several distances using both synthetic and real data. Our approach is fully automatic and can provide a numerical distance estimation for up to six meters, beyond which changes in facial distortion are not significant. The proposed method achieves an accurate estimation, with an average error below 6 cm of subject-to-camera distance for facial photographs in any frontal or lateral head pose, robust to facial hair, glasses, and partial occlusion.
Keywords
Subject-to-camera distance
Perspective distortion
Photography
Human identification
Deep learning
Transfer learning
Perspective distortion
Photography
Human identification
Deep learning
Transfer learning
Editor version
Rights
Atribución-NoComercial-SinDerivadas 3.0 España
ISSN
0957-4174