A One-Class Classification method based on Expanded Non-Convex Hulls
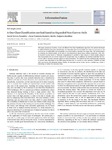
Use this link to cite
http://hdl.handle.net/2183/31809
Except where otherwise noted, this item's license is described as Atribución-NoComercial-SinDerivadas 3.0 España
Collections
- Investigación (FIC) [1679]
Metadata
Show full item recordTitle
A One-Class Classification method based on Expanded Non-Convex HullsDate
2023Citation
D. Novoa-Paradela, O. Fontenla-Romero, y B. Guijarro-Berdiñas, «A One-Class Classification method based on Expanded Non-Convex Hulls», Information Fusion, vol. 89, pp. 1-15, ene. 2023, doi: 10.1016/j.inffus.2022.07.023.
Abstract
[Abstract]: This paper presents an intuitive, robust and efficient One-Class Classification algorithm. The method developed is called OCENCH (One-class Classification via Expanded Non-Convex Hulls) and bases its operation on the construction of subdivisible and expandable non-convex hulls to represent the target class. The method begins by reducing the dimensionality of the data to two-dimensional spaces using random projections. After that, an iterative process based on Delaunay triangulations is applied to these spaces to obtain simple polygons that characterizes the non-convex shape of the normal class data. In addition, the method subdivides the non-convex hulls to represent separate regions in space if necessary. The method has been evaluated and compared to several main algorithms of the field using real data sets. In contrast to other methods, OCENCH can deal with non-convex and disjointed shapes. Finally, its execution can be carried out in a parallel way, which is interesting to reduce the execution time.
Keywords
Machine learning
One-class classification
Convex hull
Delaunay triangulation
Random projections
Ensemble learning
One-class classification
Convex hull
Delaunay triangulation
Random projections
Ensemble learning
Editor version
Rights
Atribución-NoComercial-SinDerivadas 3.0 España
ISSN
1566-2535