Improving handgun detectors with human pose classification
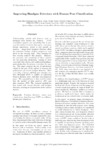
Use este enlace para citar
http://hdl.handle.net/2183/31432
A non ser que se indique outra cousa, a licenza do ítem descríbese como Atribución-NoComercial-CompartirIgual 4.0 Internacional (CC BY-NC-SA 4.0)
https://creativecommons.org/licenses/by-nc-sa/4.0/deed.es
Coleccións
Metadatos
Mostrar o rexistro completo do ítemTítulo
Improving handgun detectors with human pose classificationAutor(es)
Data
2022Cita bibliográfica
Ruiz-Santaquiteria, J., Deniz, O., Vallez, N., Velasco-Mata, A., Bueno, G. (2022) Improving handgun detectors with human pose classification. XLIII Jornadas de Automática: libro de actas, pp.1040-1047 https://doi.org/10.17979/spudc.9788497498418.1040
Resumo
[Abstract] Unfortunately, attacks with firearms such as handguns have become too common. CCTV surveillance systems can potentially help to prevent this kind of incidents, but require continuous human supervision, which is not feasible in practice. Image-based handgun detectors allow the automatic location of these weapons to send alerts to the security staff. Deep learning has been recently used for this purpose. However, the precision and sensitivity of these systems are not generally satisfactory, causing in most cases both false alarms and undetected handguns, particularly when the firearm is far from the camera. This paper proposes the use of information related to the pose of the subject to improve the performance of current handgun detectors. More concretely, a human full-body pose classifier has been developed which is capable of separating between shooting poses and other non-dangerous poses. The classified pose is then used to reduce both the number of false positives (FP) and false negatives (FN). The proposed method has been tested with several datasets and handgun detectors, showing an improvement under various metrics.
Palabras chave
Handgun detection
Human pose classification
Deep learning
CCTV surveillance
Human pose estimation
Human pose classification
Deep learning
CCTV surveillance
Human pose estimation
Versión do editor
Dereitos
Atribución-NoComercial-CompartirIgual 4.0 Internacional (CC BY-NC-SA 4.0)
https://creativecommons.org/licenses/by-nc-sa/4.0/deed.es
ISBN
978-84-9749-841-8