Machine Learning Techniques to Predict Different Levels of Hospital Care of CoVid-19
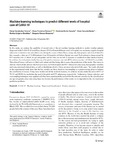
Use este enlace para citar
http://hdl.handle.net/2183/30533Colecciones
- GI-LIDIA - Artigos [65]
Metadatos
Mostrar el registro completo del ítemTítulo
Machine Learning Techniques to Predict Different Levels of Hospital Care of CoVid-19Autor(es)
Fecha
2022Cita bibliográfica
Hernández-Pereira, E., Fontenla-Romero, O., Bolón-Canedo, V. et al. Machine learning techniques to predict different levels of hospital care of CoVid-19. Appl Intell 52, 6413–6431 (2022). https://doi.org/10.1007/s10489-021-02743-2
Resumen
[Abstract] In this study, we analyze the capability of several state of the art machine learning methods to predict whether patients diagnosed with CoVid-19 (CoronaVirus disease 2019) will need different levels of hospital care assistance (regular hospital admission or intensive care unit admission), during the course of their illness, using only demographic and clinical data. For this research, a data set of 10,454 patients from 14 hospitals in Galicia (Spain) was used. Each patient is characterized by 833 variables, two of which are age and gender and the other are records of diseases or conditions in their medical history. In addition, for each patient, his/her history of hospital or intensive care unit (ICU) admissions due to CoVid-19 is available. This clinical history will serve to label each patient and thus being able to assess the predictions of the model. Our aim is to identify which model delivers the best accuracies for both hospital and ICU admissions only using demographic variables and some structured clinical data, as well as identifying which of those are more relevant in both cases. The results obtained in the experimental study show that the best models are those based on oversampling as a preprocessing phase to balance the distribution of classes. Using these models and all the available features, we achieved an area under the curve (AUC) of 76.1% and 80.4% for predicting the need of hospital and ICU admissions, respectively. Furthermore, feature selection and oversampling techniques were applied and it has been experimentally verified that the relevant variables for the classification are age and gender, since only using these two features the performance of the models is not degraded for the two mentioned prediction problems.
Palabras clave
CoVid-19
Machine learning
Supervised classification
Feature selection
Machine learning
Supervised classification
Feature selection
Descripción
Financiado para publicación en acceso aberto: Universidade da Coruña/CISUG
Versión del editor
Derechos
Atribución 4.0 Internacional