IoT Dataset Validation Using Machine Learning Techniques for Traffic Anomaly Detection
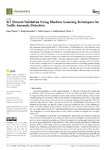
Use este enlace para citar
http://hdl.handle.net/2183/30241Colecciones
Metadatos
Mostrar el registro completo del ítemTítulo
IoT Dataset Validation Using Machine Learning Techniques for Traffic Anomaly DetectionFecha
2021Cita bibliográfica
Vigoya, L.; Fernandez, D.; Carneiro, V.; Nóvoa, F.J. IoT Dataset Validation Using Machine Learning Techniques for Traffic Anomaly Detection. Electronics 2021, 10, 2857. https://doi.org/10.3390/electronics10222857
Resumen
[Abstract] With advancements in engineering and science, the application of smart systems is increasing, generating a faster growth of the IoT network traffic. The limitations due to IoT restricted power and computing devices also raise concerns about security vulnerabilities. Machine learning-based techniques have recently gained credibility in a successful application for the detection of network anomalies, including IoT networks. However, machine learning techniques cannot work without representative data. Given the scarcity of IoT datasets, the DAD emerged as an instrument for knowing the behavior of dedicated IoT-MQTT networks. This paper aims to validate the DAD dataset by applying Logistic Regression, Naive Bayes, Random Forest, AdaBoost, and Support Vector Machine to detect traffic anomalies in IoT. To obtain the best results, techniques for handling unbalanced data, feature selection, and grid search for hyperparameter optimization have been used. The experimental results show that the proposed dataset can achieve a high detection rate in all the experiments, providing the best mean accuracy of 0.99 for the tree-based models, with a low false-positive rate, ensuring effective anomaly detection.
Palabras clave
IoT
Sensors
Dataset validation
Machine learning
Intrusion detection systems
Analysis
Metric
Algorithm design
Sensors
Dataset validation
Machine learning
Intrusion detection systems
Analysis
Metric
Algorithm design
Descripción
This article belongs to the Special Issue Sensor Network Technologies and Applications with Wireless Sensor Devices
Versión del editor
Derechos
Atribución 4.0 Internacional
ISSN
2079-9292