How Important Is Data Quality? Best Classifiers vs Best Features
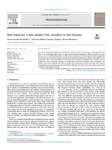
Use this link to cite
http://hdl.handle.net/2183/30051
Except where otherwise noted, this item's license is described as Atribución-NoComercial-SinDerivadas 4.0 Internacional
Collections
- Investigación (FIC) [1685]
Metadata
Show full item recordTitle
How Important Is Data Quality? Best Classifiers vs Best FeaturesDate
2021Citation
MORÁN-FERNÁNDEZ, Laura, BÓLON-CANEDO, Verónica and ALONSO-BETANZOS, Amparo, 2022. How important is data quality? Best classifiers vs best features. Neurocomputing. 22 January 2022. Vol. 470, p. 365–375. DOI 10.1016/j.neucom.2021.05.107
Abstract
[Abstract] The task of choosing the appropriate classifier for a given scenario is not an easy-to-solve question. First, there is an increasingly high number of algorithms available belonging to different families. And also there is a lack of methodologies that can help on recommending in advance a given family of algorithms for a certain type of datasets. Besides, most of these classification algorithms exhibit a degradation in the performance when faced with datasets containing irrelevant and/or redundant features. In this work we analyze the impact of feature selection in classification over several synthetic and real datasets. The experimental results obtained show that the significance of selecting a classifier decreases after applying an appropriate preprocessing step and, not only this alleviates the choice, but it also improves the results in almost all the datasets tested.
Keywords
Feature selection
Filters
Preprocessing
High dimensionality
Classification
Data análisis
Filters
Preprocessing
High dimensionality
Classification
Data análisis
Description
Financiado para publicación en acceso aberto: Universidade da Coruña/CISUG
Editor version
Rights
Atribución-NoComercial-SinDerivadas 4.0 Internacional