Scalable Feature Selection Using ReliefF Aided by Locality-Sensitive Hashing
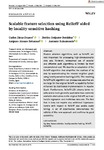
Use this link to cite
http://hdl.handle.net/2183/28846
Except where otherwise noted, this item's license is described as Atribución-NoComercial 4.0 Internacional
Collections
- GI-LIDIA - Artigos [50]
Metadata
Show full item recordTitle
Scalable Feature Selection Using ReliefF Aided by Locality-Sensitive HashingDate
2021Citation
Eiras‐Franco C, Guijarro‐Berdiñas B, Alonso‐Betanzos A, Bahamonde A. Scalable feature selection using ReliefF aided by locality‐sensitive hashing. Int J Intell Syst. 2021;36:6161‐6179. https://doi.org/10.1002/int.22546
Abstract
[Abstract] Feature selection algorithms, such as ReliefF, are very important for processing high-dimensionality data sets. However, widespread use of popular and effective such algorithms is limited by their computational cost. We describe an adaptation of the ReliefF algorithm that simplifies the costliest of its step by approximating the nearest neighbor graph using locality-sensitive hashing (LSH). The resulting ReliefF-LSH algorithm can process data sets that are too large for the original ReliefF, a capability further enhanced by distributed implementation in Apache Spark. Furthermore, ReliefF-LSH obtains better results and is more generally applicable than currently available alternatives to the original ReliefF, as it can handle regression and multiclass data sets. The fact that it does not require any additional hyperparameters with respect to ReliefF also avoids costly tuning. A set of experiments demonstrates the validity of this new approach and confirms its good scalability.
Keywords
Big data
Feature selection
Locality-sensitive hashing
ReliefF
Scalability
Feature selection
Locality-sensitive hashing
ReliefF
Scalability
Description
Financiado para publicación en acceso aberto: Universidade da Coruña/CISUG
Editor version
Rights
Atribución-NoComercial 4.0 Internacional