SOPRENE: Assessment of the Spanish Armada’s Predictive Maintenance Tool for Naval Assets
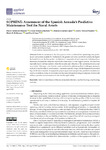
Use este enlace para citar
http://hdl.handle.net/2183/28448
Excepto si se señala otra cosa, la licencia del ítem se describe como Atribución 4.0 Internacional (CC BY 4.0)
Colecciones
- Investigación (FIC) [1679]
Metadatos
Mostrar el registro completo del ítemTítulo
SOPRENE: Assessment of the Spanish Armada’s Predictive Maintenance Tool for Naval AssetsAutor(es)
Fecha
2021Cita bibliográfica
Fernández-Barrero, D.; Fontenla-Romero, O.; Lamas-López, F.; Novoa-Paradela, D.; R-Moreno, M.D.; Sanz, D. SOPRENE: Assessment of the Spanish Armada’s Predictive Maintenance Tool for Naval Assets. Appl. Sci. 2021, 11, 7322. https://doi.org/10.3390/app11167322
Resumen
[Abstract] Predictive maintenance has lately proved to be a useful tool for optimizing costs, performance and systems availability. Furthermore, the greater and more complex the system, the higher the benefit but also the less applied: Architectural, computational and complexity limitations have historically ballasted the adoption of predictive maintenance on the biggest systems. This has been especially true in military systems where the security and criticality of the operations do not accept uncertainty. This paper describes the work conducted in addressing these challenges, aiming to evaluate its applicability in a real scenario: It presents a specific design and development for an actual big and diverse ecosystem of equipment, proposing an semi-unsupervised predictive maintenance system. In addition, it depicts the solution deployment, test and technological adoption of real-world military operative environments and validates the applicability.
Palabras clave
Predictive maintenance
Behavioural anomaly detection
Machine learning
Deep learning
Warships
Behavioural anomaly detection
Machine learning
Deep learning
Warships
Versión del editor
Derechos
Atribución 4.0 Internacional (CC BY 4.0)