Análisis de técnicas de aumento de datos y entrenamiento en YOLOv3 para detección de objetos en imágenes RGB y TIR del UMA-SAR Dataset
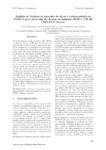
View/ Open
Use this link to cite
http://hdl.handle.net/2183/28378
Except where otherwise noted, this item's license is described as Atribución-NoComercial-CompartirIgual 4.0 Internacional
https://creativecommons.org/licenses/by-nc-sa/4.0/deed.es
Collections
Metadata
Show full item recordTitle
Análisis de técnicas de aumento de datos y entrenamiento en YOLOv3 para detección de objetos en imágenes RGB y TIR del UMA-SAR DatasetAuthor(s)
Date
2021Citation
Galán-Cuenca, A., Vázquez-Martín, R., Mandow, A., Morales, J., García-Cerezo, A. Análisis de técnicas de aumento de datos y entrenamiento en YOLOv3 para detección de objetos en imágenes RGB y TIR del UMA-SAR Dataset. En XLII Jornadas de Automática: libro de actas. Castelló, 1-3 de septiembre de 2021 (pp. 686-694). DOI capítulo: https://doi.org/10.17979/spudc.9788497498043.686 DOI libro: https://doi.org/10.17979/spudc.9788497498043
Abstract
[Resumen] El uso de imágenes de los espectros visible (RGB) e infrarrojo térmico (TIR) para la detección de objetos puede resultar crucial en aplicaciones donde
las condiciones de visibilidad están limitadas, como la robótica para búsqueda y rescate en catástrofes. Para ello resulta beneficioso analizar cómo las técnicas de aprendizaje profundo basadas en redes neuronales convolucionales (CNN) pueden aplicarse a ambas modalidades. En este artículo se
analizan diferentes configuraciones y parámetros para el entrenamiento de CNN tanto para imágenes térmicas como para imágenes equivalentes del
espectro visible. En concreto, se aborda el problema del sobre-entrenamiento para determinar una configuración eficaz de técnicas de aumento de datos
y parada temprana. El caso de estudio se ha realizado con la red de código abierto YOLOv3, pre-entrenada con el dataset RGB COCO y optimizada
(o re-entrenada) con el conjunto público de datos UMA-SAR dataset, que incluye pares de imágenes RGB y TIR obtenidas en ejercicios realistas de rescate. [Abstract] The combination of imaging of visible (RGB) and thermal infrared (TIR) modalities can be crucial for object detection in applications where visibility conditions
are limited, such as search and rescue robotics. For this, it is beneficial to analyze how deep learning techniques based on convolutional neural networks (CNN) can be applied to these modalities. This article discusses different settings and parameters for CNN training for two equivalent sets of thermal and RGB images. Specifically, we address the problem of overfitting and determine an effective configuration of data augmentation and early stop techniques. The case study has been carried out with the open source network YOLOv3, pre-trained with the RGB COCO dataset, and optimised with the UMA-SAR dataset, which includes pairs of RGB and TIR images obtained in realistic rescue exercises.
Keywords
Visión por computador
Aprendizaje profundo
Redes neuronales convolucionales
YOLO
Imágenes térmicas
Computer vision
Deep learning
Convolutional neural networks
Thermal imaging
Aprendizaje profundo
Redes neuronales convolucionales
YOLO
Imágenes térmicas
Computer vision
Deep learning
Convolutional neural networks
Thermal imaging
Editor version
Rights
Atribución-NoComercial-CompartirIgual 4.0 Internacional
https://creativecommons.org/licenses/by-nc-sa/4.0/deed.es
ISBN
978-84-9749-804-3