Detección de anomalías en la red empleando técnicas de machine learning
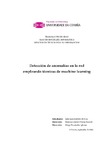
Use este enlace para citar
http://hdl.handle.net/2183/26827
Excepto si se señala otra cosa, la licencia del ítem se describe como Atribución-NoComercial-SinDerivadas 3.0 España
Colecciones
Metadatos
Mostrar el registro completo del ítemTítulo
Detección de anomalías en la red empleando técnicas de machine learningAutor(es)
Directores
Nóvoa Manuel, Francisco JavierFernández Iglesias, Diego
Fecha
2020-09Centro/Dpto/Entidad
Enxeñaría informática, Grao enDescripción
Traballo fin de grao (UDC.FIC). Enxeñaría informática. Curso 2019/2020Resumen
[Resumen]
En el ámbito de las redes de comunicación, una anomalía puede ser definida como una
variación repentina del comportamiento habitual. Esto incluye tanto eventos fortuitos y bien
intencionados, como ataques deliberados pensados para comprometer la disponibilidad de
la red. En ambos casos, es esencial detectar rápido estas anomalías para poder reaccionar a
tiempo.
En los últimos años, las técnicas de machine learning se han ido haciendo un lugar como
alternativa a los sistemas tradicionales de detección de intrusiones basados en políticas, puesto
que acarrean una serie de ventajas. Entre ellas, las técnicas de machine learning posibilitan el
desarrollo de algoritmos no paramétricos, adaptativos a nuestra red y a sus modificaciones, y
portables entre aplicaciones.
En este trabajo se revisan diferentes alternativas de machine learning, a medida que modelamos
un dataset etiquetado con el tráfico de una red corporativa agregado en flujos de
red. El proceso supone examinar minuciosamente el conjunto de datos, y seleccionar los más
relevantes según nuestro dominio y las técnicas que empleamos. Después, buscamos la combinación
idónea de parámetros para optimizar el rendimiento de los algoritmos y compararlos
entre sí, de manera que podamos averiguar cuál es el mejor de forma objetiva.
También se barajan diferentes opciones en materia de computación distribuida para el
despliegue, teniendo como objetivo la predicción en tiempo real de amenazas, y como requisito
que sea posible el procesamiento de datos con características de Big Data. A fin de conseguir
esto, se definen varias configuraciones de despliegue, y se emplean diferentes tecnologías que
permiten emular el escenario descrito de predicción en tiempo real. En esta parte empleamos
como infraestructura un cluster de diez máquinas. Por último, la solución que se despliega
está basada en el mejor modelo obtenido. [Abstract]
A network anomaly can be defined as a variation of the regular behaviour of the network.
That includes both unfortunate unintended events, and deliberate attacks planned to compromise
the network’s availability. In both cases, it is essential to be able to detect those quickly
so we can react in time.
In the past few years, machine learning has been slowly taking its place as an alternative
to policies-based traditional intrusion detection systems, as it presents quite a few advantages.
Amongst them, machine learning techniques allow the development of non-parametric algorithms,
adaptative to our network and its modifications, and portable across applications.
In this paper we go through different machine learning and Big Data alternatives, as we
model a labelled dataset containing the traffic of a corporative network aggregated in network
flows. The process entails careful examination of the dataset, and the selection of the
most relevant subset of data according to our domain and the employed techniques. Then we
look for the best parameters combination to optimize the algorithms’ performance, and we
compare them to find out which one is the best in an objective way.
We also consider different options for deployment involving distributed computing, having
as goal the prediction of network threats in real-time, and as requisite being able to process
Big Data. For achieving this, we define several deployment configurations, and use different
technologies so we can emulate the real-time prediction scenario that we just described. In
this phase we rely on a cluster with ten machines as our infrastructure. Lastly, the solution
deployed is based on the best model obtained.
Palabras clave
Seguridad de red
Computación distribuida
Flujo de red
Anomalía
Clasificación
Machine learning
IDS
Network security
Distributed computing
Big Data
Network flow
Anomaly
Classification
Random forest
Naïve Bayes
Deep neural networks
Computación distribuida
Flujo de red
Anomalía
Clasificación
Machine learning
IDS
Network security
Distributed computing
Big Data
Network flow
Anomaly
Classification
Random forest
Naïve Bayes
Deep neural networks
Derechos
Atribución-NoComercial-SinDerivadas 3.0 España