Perturbation-Theory Machine Learning (PTML) Multilabel Model of the ChEMBL Dataset of Preclinical Assays for Antisarcoma Compounds
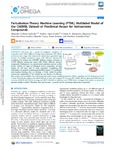
Ver/Abrir
Use este enlace para citar
http://hdl.handle.net/2183/26747Colecciones
- Investigación (FIC) [1685]
Metadatos
Mostrar el registro completo del ítemTítulo
Perturbation-Theory Machine Learning (PTML) Multilabel Model of the ChEMBL Dataset of Preclinical Assays for Antisarcoma CompoundsAutor(es)
Fecha
2020-10-14Cita bibliográfica
Cabrera-Andrade A, López-Cortés A, Munteanu CR, et al. Perturbation-Theory Machine Learning (PTML) Multilabel Model of the ChEMBL Dataset of Preclinical Assays for Antisarcoma Compounds. ACS Omega. 2020; 5(42):27211-27220
Resumen
[Abstract]
Sarcomas are a group of malignant neoplasms of connective tissue with a different etiology than carcinomas. The efforts to discover new drugs with antisarcoma activity have generated large datasets of multiple preclinical assays with different experimental conditions. For instance, the ChEMBL database contains outcomes of 37,919 different antisarcoma assays with 34,955 different chemical compounds. Furthermore, the experimental conditions reported in this dataset include 157 types of biological activity parameters, 36 drug targets, 43 cell lines, and 17 assay organisms. Considering this information, we propose combining perturbation theory (PT) principles with machine learning (ML) to develop a PTML model to predict antisarcoma compounds. PTML models use one function of reference that measures the probability of a drug being active under certain conditions (protein, cell line, organism, etc.). In this paper, we used a linear discriminant analysis and neural network to train and compare PT and non-PT models. All the explored models have an accuracy of 89.19–95.25% for training and 89.22–95.46% in validation sets. PTML-based strategies have similar accuracy but generate simplest models. Therefore, they may become a versatile tool for predicting antisarcoma compounds.
Palabras clave
Assays
Chemical specificity
Molecular modeling
Mathematical methods
Bioactivity
Chemical specificity
Molecular modeling
Mathematical methods
Bioactivity
Versión del editor
Derechos
This is an open access article published under an ACS AuthorChoice License, which permits
copying and redistribution of the article or any adaptations for non-commercial purposes.
ISSN
2470-1343