Enhancing Retinal Blood Vessel Segmentation through Self-Supervised Pre-Training
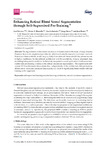
Use this link to cite
http://hdl.handle.net/2183/26575
Except where otherwise noted, this item's license is described as Atribución 4.0 International (CC BY 4.0)
Collections
- Investigación (FIC) [1685]
Metadata
Show full item recordTitle
Enhancing Retinal Blood Vessel Segmentation through Self-Supervised Pre-TrainingDate
2020-08-25Citation
Morano, J.; Hervella, Á.S.; Barreira, N.; Novo, J.; Rouco, J. Enhancing Retinal Blood Vessel Segmentation through Self-Supervised Pre-Training. Proceedings 2020, 54, 44. https://doi.org/10.3390/proceedings2020054044
Abstract
[Abstract]
The segmentation of the retinal vasculature is fundamental in the study of many diseases. However, its manual completion is problematic, which motivates the research on automatic methods. Nowadays, these methods usually employ Fully Convolutional Networks (FCNs), whose success is highly conditioned by the network architecture and the availability of many annotated data, something infrequent in medicine. In this work, we present a novel application of self-supervised multimodal pre-training to enhance the retinal vasculature segmentation. The experiments with diverse FCN architectures demonstrate that, independently of the architecture, this pre-training allows one to overcome annotated data scarcity and leads to significantly better results with less training on the target task.
Keywords
Self-supervised learning
Transfer learning
Multimodal
Retinal vasculature segmentation
Transfer learning
Multimodal
Retinal vasculature segmentation
Editor version
Rights
Atribución 4.0 International (CC BY 4.0)
ISSN
2504-3900