Regression Tree Based Explanation for Anomaly Detection Algorithm
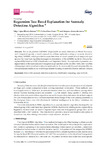
Use este enlace para citar
http://hdl.handle.net/2183/26501Colecciones
- Investigación (FIC) [1634]
Metadatos
Mostrar el registro completo del ítemTítulo
Regression Tree Based Explanation for Anomaly Detection AlgorithmFecha
2020-08-18Cita bibliográfica
Botana, I.L.-R.; Eiras-Franco, C.; Alonso-Betanzos, A. Regression Tree Based Explanation for Anomaly Detection Algorithm. Proceedings 2020, 54, 7. https://doi.org/10.3390/proceedings2020054007
Resumen
[Abstract]
This work presents EADMNC (Explainable Anomaly Detection on Mixed Numerical and Categorical spaces), a novel approach to address explanation using an anomaly detection algorithm, ADMNC, which provides accurate detections on mixed numerical and categorical input spaces. Our improved algorithm leverages the formulation of the ADMNC model to offer pre-hoc explainability based on CART (Classification and Regression Trees). The explanation is presented as a segmentation of the input data into homogeneous groups that can be described with a few variables, offering supervisors novel information for justifications. To prove scalability and interpretability, we list experimental results on real-world large datasets focusing on network intrusion detection domain.
Palabras clave
XAI
CART
Anomaly detection
Scalability
Distributed computing
Apache Spark
CART
Anomaly detection
Scalability
Distributed computing
Apache Spark
Versión del editor
Derechos
Atribución 4.0 Internacional
ISSN
2504-3900