Network Anomaly Detection Using Machine Learning Techniques
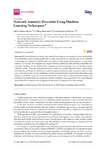
Use este enlace para citar
http://hdl.handle.net/2183/26422Colecciones
Metadatos
Mostrar el registro completo del ítemTítulo
Network Anomaly Detection Using Machine Learning TechniquesFecha
2020-08-19Cita bibliográfica
Estévez-Pereira, J.J.; Fernández, D.; Novoa, F.J. Network Anomaly Detection Using Machine Learning Techniques. Proceedings 2020, 54, 8. https://doi.org/10.3390/proceedings2020054008
Resumen
[Abstract]
While traditional network security methods have been proven useful until now, the flexibility of machine learning techniques makes them a solid candidate in the current scene of our networks. In this paper, we assess how well the latter are capable of detecting security threats in a corporative network. To that end, we configure and compare several models to find the one which fits better with our needs. Furthermore, we distribute the computational load and storage so we can handle extensive volumes of data. The algorithms that we use to create our models, Random Forest, Naive Bayes, and Deep Neural Networks (DNN), are both divergent and tested in other papers in order to make our comparison richer. For the distribution phase, we operate with Apache Structured Streaming, PySpark, and MLlib. As for the results, it is relevant to mention that our dataset has been found to be effectively modelable with just a reduced number of features. Finally, given the outcomes obtained, we find this line of research encouraging and, therefore, this approach worth pursuing.
Palabras clave
Machine learning
IDS
Network security
Distributed computing
Network flow
IDS
Network security
Distributed computing
Network flow
Versión del editor
Derechos
Atribución 4.0 Internacional
ISSN
2504-3900