Novel and Diverse Recommendations by Leveraging Linear Models with User and Item Embeddings
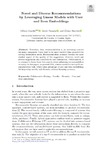
Use este enlace para citar
http://hdl.handle.net/2183/25591Colecciones
- Investigación (FIC) [1576]
Metadatos
Mostrar el registro completo del ítemTítulo
Novel and Diverse Recommendations by Leveraging Linear Models with User and Item EmbeddingsFecha
2020-04-08Cita bibliográfica
Landin A., Parapar J., Barreiro Á. (2020) Novel and Diverse Recommendations by Leveraging Linear Models with User and Item Embeddings. In: Jose J. et al. (eds) Advances in Information Retrieval. ECIR 2020. Lecture Notes in Computer Science, vol 12036. Springer, Cham. https://doi.org/10.1007/978-3-030-45442-5_27
Resumen
[Abstract] Nowadays, item recommendation is an increasing concern for many companies. Users tend to be more reactive than proactive for solving information needs. Recommendation accuracy became the most studied aspect of the quality of the suggestions. However, novel and diverse suggestions also contribute to user satisfaction. Unfortunately, it is common to harm those two aspects when optimizing recommendation accuracy. In this paper, we present EER, a linear model for the top-N recommendation task, which takes advantage of user and item embeddings for improving novelty and diversity without harming accuracy.
Palabras clave
Collaborative filtering
Novelty
Diversity
User and item embeddings
Novelty
Diversity
User and item embeddings
Versión del editor
Derechos
© Springer Nature Switzerland AG 2020
ISSN
0302-9743
ISBN
9783030454418 9783030454425