Fully automatic multi-temporal land cover classification using Sentinel-2 image data
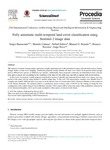
Use this link to cite
http://hdl.handle.net/2183/24597
Except where otherwise noted, this item's license is described as Atribución-NoComercial-SinDerivadas 4.0 España
Collections
Metadata
Show full item recordTitle
Fully automatic multi-temporal land cover classification using Sentinel-2 image dataAuthor(s)
Date
2019Citation
Baamonde, Sergio, et al. Fully automatic multi-temporal land cover classification using Sentinel-2 image data. Procedia Computer Science, 2019, vol. 159, p. 650-657.
Abstract
[Abstract] The analysis of remote sensing images represents a highly important issue to be performed in many relevant fields such as climate change studies or land cover mapping. Traditional proposals usually identify the land cover classes from general related groups such as different tree species or different crop varieties. Additionally, these proposals commonly use information from a precise time span or season, not accounting for the variability of the data over the entire year, specially in regions with several seasons.
In this work, we propose a multi-temporal classification system to identify and represent diverse land cover classes over any period of the entire year by using Sentinel-2 satellite image data. To this end, 526 representative samples were labelled from 5 complex and variable different land cover types over the Special Area of Conservation (SAC) Betanzos-Mandeo in the northwest of the Iberian Peninsula. The method achieves a satisfactory mean accuracy value of 84.0% for the testing set using the best configuration with a radial Support Vector Machine classifier. This system will be used in the study of the population connectivity of two threatened herptiles, but it can be easily extended to other species of interest in the future.
Keywords
Remote sensing
Sentinel-2
Land cover classification
Machine learning
Sentinel-2
Land cover classification
Machine learning
Editor version
Rights
Atribución-NoComercial-SinDerivadas 4.0 España
ISSN
1877-0509