Servet: A Benchmark Suite for Autotuning on Multicore Clusters
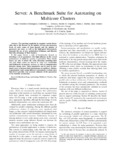
Use this link to cite
http://hdl.handle.net/2183/22670Collections
- Investigación (FIC) [1634]
Metadata
Show full item recordTitle
Servet: A Benchmark Suite for Autotuning on Multicore ClustersAuthor(s)
Date
2010-05-24Citation
GONZÁLEZ-DOMÍNGUEZ, Jorge, et al. Servet: A benchmark suite for autotuning on multicore clusters. En 2010 IEEE International Symposium on Parallel & Distributed Processing (IPDPS). IEEE, 2010. p. 1-9.
Abstract
[Abstract] MapReduce is a powerful tool for processing large data sets used by many applications running in distributed environments. However, despite the increasing number of computationally intensive problems that require low-latency communications, the adoption of MapReduce in High Performance Computing (HPC) is still emerging. Here languages based on the Partitioned Global Address Space (PGAS) programming model have shown to be a good choice for implementing parallel applications, in order to take advantage of the increasing number of cores per node and the programmability benefits achieved by their global memory view, such as the transparent access to remote data. This paper presents the first PGAS-based MapReduce implementation that uses the Unified Parallel C (UPC) language, which (1) obtains programmability benefits in parallel programming, (2) offers advanced configuration options to define a customized load distribution for different codes, and (3) overcomes performance penalties and bottlenecks that have traditionally prevented the deployment of MapReduce applications in HPC. The performance evaluation of representative applications on shared and distributed memory environments assesses the scalability of the presented MapReduce framework, confirming its suitability.
Keywords
Multicore processing
Hardware
Computer architecture
Delay
Parameter estimation
Libraries
Bandwidth
Clustering
Algorithms
Parallel processing
Parallel architectures
Hardware
Computer architecture
Delay
Parameter estimation
Libraries
Bandwidth
Clustering
Algorithms
Parallel processing
Parallel architectures
Description
This is a post-peer-review, pre-copyedit version of an article published in 2010 IEEE International Symposium on Parallel & Distributed Processing (IPDPS). Proceedings. The final authenticated version is available online at: http://dx.doi.org/10.1109/IPDPS.2010.5470358.