Gastrointestinal Spatiotemporal mRNA Expression of Ghrelin vs Growth Hormone Receptor and New Growth Yield Machine Learning Model Based on Perturbation Theory
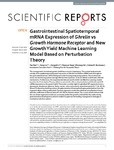
Ver/ abrir
Use este enlace para citar
http://hdl.handle.net/2183/22609Coleccións
- GI-RNASA - Artigos [193]
Metadatos
Mostrar o rexistro completo do ítemTítulo
Gastrointestinal Spatiotemporal mRNA Expression of Ghrelin vs Growth Hormone Receptor and New Growth Yield Machine Learning Model Based on Perturbation TheoryAutor(es)
Data
2016-07-27Cita bibliográfica
Ran T, Liu Y, Li H, et al. Gastrointestinal spatiotemporal mRNA expression of ghrelin vs growth hormone receptor and new growth yield machine learning model based on perturbation theory. Sci Rep. 2016; 6:30174
Resumo
[Abstract] The management of ruminant growth yield has economic importance. The current work presents a study of the spatiotemporal dynamic expression of Ghrelin and GHR at mRNA levels throughout the gastrointestinal tract (GIT) of kid goats under housing and grazing systems. The experiments show that the feeding system and age affected the expression of either Ghrelin or GHR with different mechanisms. Furthermore, the experimental data are used to build new Machine Learning models based on the Perturbation Theory, which can predict the effects of perturbations of Ghrelin and GHR mRNA expression on the growth yield. The models consider eight longitudinal GIT segments (rumen, abomasum, duodenum, jejunum, ileum, cecum, colon and rectum), seven time points (0, 7, 14, 28, 42, 56 and 70 d) and two feeding systems (Supplemental and Grazing feeding) as perturbations from the expected values of the growth yield. The best regression model was obtained using Random Forest, with the coefficient of determination R2 of 0.781 for the test subset. The current results indicate that the non-linear regression model can accurately predict the growth yield and the key nodes during gastrointestinal development, which is helpful to optimize the feeding management strategies in ruminant production system.
Palabras chave
Abomasum
Animal feed
Gastrointestinal tract
Gene expression
Ghrelin
Goats
Machine learning
RNA messenger
Receptors
Rumen
Animal feed
Gastrointestinal tract
Gene expression
Ghrelin
Goats
Machine learning
RNA messenger
Receptors
Rumen
Versión do editor
Dereitos
Atribución 3.0 España
ISSN
2045-2322