Optimization of Real-World MapReduce Applications With Flame-MR: Practical Use Cases
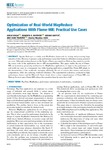
Use este enlace para citar
http://hdl.handle.net/2183/21731Coleccións
- Investigación (FIC) [1656]
Metadatos
Mostrar o rexistro completo do ítemTítulo
Optimization of Real-World MapReduce Applications With Flame-MR: Practical Use CasesData
2018-11-12Cita bibliográfica
J. Veiga et al, "Optimization of real-world MapReduce applications with Flame-MR: practical use cases," IEEE Access, vol. 6, pp. 69750-69762, 2018.
Resumo
[Abstract] Apache Hadoop is a widely used MapReduce framework for storing and processing large amounts of data. However, it presents some performance issues that hinder its utilization in many practical use cases. Although existing alternatives like Spark or Hama can outperform Hadoop, they require to rewrite the source code of the applications due to API incompatibilities. This paper studies the use of Flame-MR, an in-memory processing architecture for MapReduce applications, to improve the performance of real-world use cases in a transparent way while keeping application compatibility. Flame-MR adapts to the characteristics of the workloads, managing efficiently the use of custom data formats and iterative computations, while also reducing workload imbalance. The experimental evaluation, conducted in high performance clusters and the Microsoft Azure cloud, shows a clear outperformance of Flame-MR over Hadoop. In most cases, Flame-MR reduces the execution times by more than a half.
Palabras chave
Data visualization
Optimization
Sparks
Data models
Data mining
Acceleration
Benchmark testing
Optimization
Sparks
Data models
Data mining
Acceleration
Benchmark testing
Versión do editor
ISSN
2169-3536