Integrative Multi-Omics Data-Driven Approach for Metastasis Prediction in Cancer
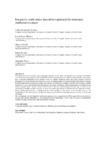
View/ Open
Use this link to cite
http://hdl.handle.net/2183/21553Collections
Metadata
Show full item recordTitle
Integrative Multi-Omics Data-Driven Approach for Metastasis Prediction in CancerDate
2018Citation
Fernández-Lozano C, Liñares Blanco J, Gestal M, et al. Integrative multi-omics data-driven approach for metastasis prediction in cancer. En: Proceedings of the First International Conference on Data Science, E-learning and Information Systems; 2018, Oct 1-2, Madrid. New York, NY: ACM; 2018 (ACM International Conference Proceeding Series)
Abstract
[Abstract]
Nowadays biomedical research is generating huge amounts of omic data, covering all levels of genetic information from nucleotide sequencing to protein metabolism. In the beginning, data were analyzed independently losing a great deal of essential information in the models. Even so, complex metabolic routes and genetic diseases could be determined. In the last decade, there has been an ever-increasing number of research projects that follow a systemic biological approach by integrating multiple omic datasets obtaining more complex, powerful and informative models that provide a deeper knowledge about the genotype-phenotype interactions.
These models greatly contributed to the study of complex multi-factorial diseases such as cancer. The onset and development of any type of cancer can be influenced by multiple variables. Integrate as many as possible omic datasets is therefore the best approach to extract all the underlying knowledge. A significant factor in the mortality of this disease is the metastatic process. The identification of the factors involved in this cell behavior may be helpful in the diagnosis and hopefully in the disease prevention.
The development of novel integrative multiomics approaches is an opportunity to fill the gaps between our ability to generate data and the difficulties to understand the biology behind them. In this work we propose a methodology pipeline for analyze multi-omics data using machine learning.
Keywords
Multi-Omics
Cancer
Data-driven
Modelling
Data integration
Machine learning
Prediction
Data fusion
Cancer
Data-driven
Modelling
Data integration
Machine learning
Prediction
Data fusion
Editor version
ISBN
978-1-4503-6536-9