Prediction of Nucleoitide Binding Peptides Using Star Graph Topological Índices
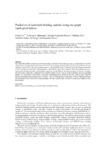
View/ Open
Use this link to cite
http://hdl.handle.net/2183/17456Collections
- INIBIC-RNASA-IMEDIR - Artigos [46]
- GI-RNASA - Artigos [193]
Metadata
Show full item recordTitle
Prediction of Nucleoitide Binding Peptides Using Star Graph Topological ÍndicesAuthor(s)
Date
2015-08-05Citation
Liu Y, Munteanu CR, Fernández Blanco E, Tan Z, Santos del Riego A, Pazos A. Prediction of nucleoitide binding peptides using star graph topological indices. Mol Inform. 2015;34(11-12):736-741
Abstract
[Abstract] The nucleotide binding proteins are involved in many important cellular processes, such as transmission of genetic information or energy transfer and storage. Therefore, the screening of new peptides for this biological function is an important research topic. The current study proposes a mixed methodology to obtain the first classification model that is able to predict new nucleotide binding peptides, using only the amino acid sequence. Thus, the methodology uses a Star graph molecular descriptor of the peptide sequences and the Machine Learning technique for the best classifier. The best model represents a Random Forest classifier based on two features of the embedded and non-embedded graphs. The performance of the model is excellent, considering similar models in the field, with an Area Under the Receiver Operating Characteristic Curve (AUROC) value of 0.938 and true positive rate (TPR) of 0.886 (test subset). The prediction of new nucleotide binding peptides with this model could be useful for drug target studies in drug development.
Keywords
QSAR
Nucleotide binding proteins
Star Graph
Topological indice
Nucleotide binding proteins
Star Graph
Topological indice
Editor version
Rights
This is the peer reviewed version of the article which has been published in final form at Wiley Online Library. This article may be used for non-commercial purposes in accordance with Wiley Terms and Conditions for self-archiving.